Resources - Blog
Rule-Based Repricing vs. Algorithmic Repricing: What’s the Difference?
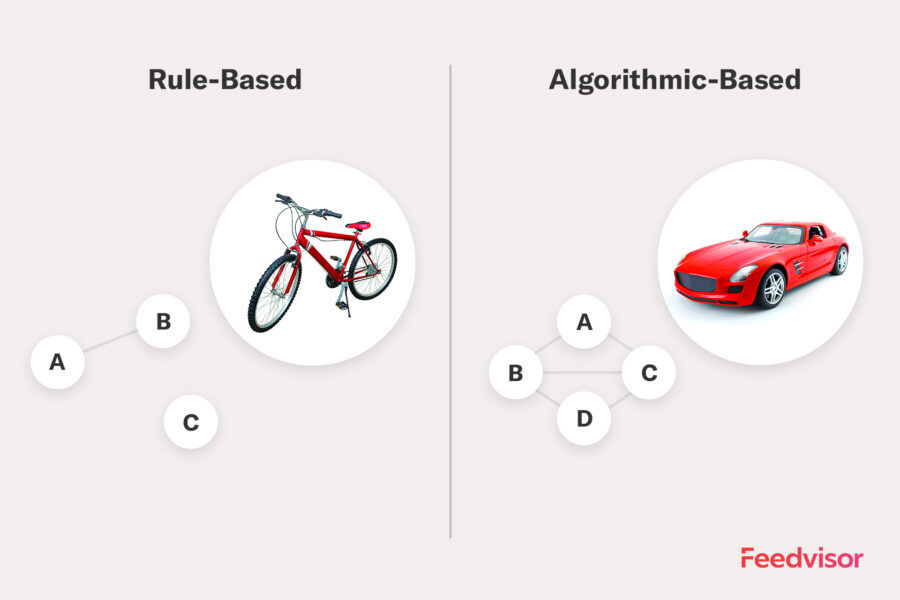
Stay on top of the latest e-commerce and marketplace trends.
Manual, Rule-Based, and Algorithmic Repricing
If you’re like many Amazon sellers, you heard the terms “algorithmic” repricer, rule-based repricer, and manual repricer, but have you ever wondered what they really mean? And how each can function to optimize your business’s growth?
The Basics of Repricing
Let’s first begin with a quick overview of the three types of repricing: there’s manual, rule-based, and algorithmic.
The Pitfalls of Manual Repricing
Manual repricing means just that: you have to manually set the prices on each of your ASINs. This is usually how Amazon sellers start out. It’s fine at the beginning, but quickly becomes unmanageable as your inventory starts expanding — along with consumer and shipping demands.
Rule-Based Repricing Limitations
Larger sellers tend to use a repricing software to automate the process, but the types of repricers out there vary significantly. Rule-based repricers involve the user choosing a blanket business rule, such as “always price x amount below the competitor.” Unfortunately for Amazon third-party sellers, many of these repricers have started branding themselves as “algorithmic” when in fact, their software consists of a couple of rules bundled together. Some repricers are still built on the false premise that having the lowest price on the market is the only way to compete successfully, and other repricers maintain constant differences; however, none of them are comparable to a learning algorithm that keeps optimizing its standing in comparison to competitors by using historical data.
Following a blind, constant rule frequently leads to price wars, meaning you’re entering a profit-sucking race to the bottom. Moreover, rule-based repricers lack the flexibility and intelligence to learn from the multitude of seller and market variables that exist. For example, if you’re an FBA seller with high feedback scores and a low order defect rate, you might not need to lower your prices in order to win the Buy Box. Sometimes, you can even raise your prices without negatively impacting your sales.
Algorithmic Repricing: The Game-Changer
That’s where algorithmic repricing comes in. When you have an algorithmic repricer, you don’t need to worry about your software leaving money on the table. A good algorithmic repricer uses millions of current and historical data points to set the optimal price for the given competitive landscape. Optimal price is not necessarily the lowest in the market; for example, the algorithm may find that it can obtain for you 50% Buy Box share with a one dollar profit margin, and that would be preferable to 100% Buy Box share with a one cent margin (assuming your goal is profit).
Artificial intelligence algorithms often have two main components: predictive (machine-learning) models that rely on historical data, and an action selection component that utilizes the data model and optimizes the system’s future behavior in expectation. Feedvisor’s predictive models analyze terabytes of data of competitors’ past actions (their price changes and timing, whether they obtained the Buy Box, etc.) in order to predict their future behavior: how their repricer responds to our price changes, what is their floor price, and so on. Rudimentary repricers are particularly exploitable: because they behave according to predefined rules, their behavior is easily anticipated by our repricer.
These models feed into the algorithmic repricer’s price selection process, which is composed of two distinct stages. First, it uses recent historical data (market prices, Buy Box winners, which of the competitors is currently active, etc.) to generate the expected profit curve as a function of the price under the current market state. Then, it calculates the optimal price according to that curve. The second stage predicts how this price change will affect the market state (i.e., how competitors will respond) in the next time period. The predictive models are used to generate a probability distribution over future market states, and for each state the algorithm assesses the expected profit and sales velocity obtainable from that point on. Furthermore, because achieving unit profit margin is a part of the algorithm’s objective, it also considers the possibility of increasing the price if (based on predictive models) it anticipates that competitors will follow.
Together, these pieces of information let the algorithm select the price point that is optimal for the client’s business needs (that is, likely to generate the highest future profit, under sales volume requirements), taking into account the range of possible competitors’ responses, potential price wars, and opportunities for reverse price wars (in which competitors follow each other with price increases).
Final Thoughts
With rule-based repricers, the burden falls on you to design the rules. In some cases, the result might be OK, but in others, it might be far from optimal. Algorithmic repricing exploits data and machine-learning models to optimize your business targets; algorithmic software constantly seeks the price point that maximizes your bottom line given current and projected market conditions. The algorithm may be complex, but the mission is simple: to keep your profits high and your business growing.
Learn what Feedvisor can do for your business.
When you partner with Feedvisor, you automatically receive access to our true, AI-driven technology and hands-on team of e-commerce experts. Contact one of our team members today to learn more about our end-to-end solution for brands and large sellers on Amazon, Walmart, and e-marketplaces.