Resources - Blog
Technology Series: Feedvisor’s Trade Intelligence Network
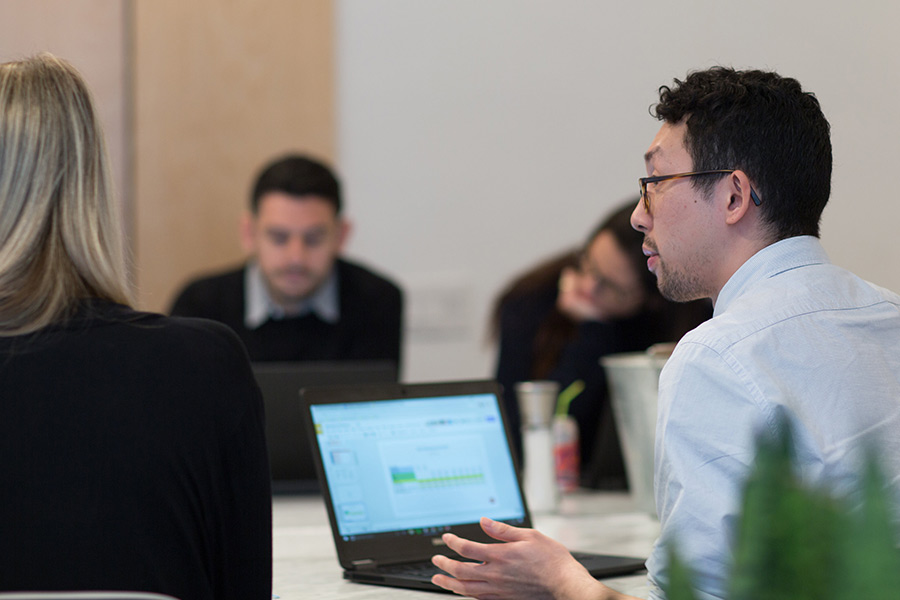
Stay on top of the latest e-commerce and marketplace trends.
This is the first blog post in a series that will focus on Feedvisor’s AI platform, data-driven intelligence, and machine-learning algorithms. The series will illustrate various ways in which our technology assists our customers in their e-commerce activities and challenges, with Amazon at the center. The series will be led by Feedvisor’s CTO and Co-founder, Eyal Lanxner.
Today, more than ever, there is a tremendous amount of commerce data generated by electronic marketplaces and websites. Together with this era’s big data technologies, scalable computing power, and advanced machine-learning methods, the business value produced from the data can be taken to new levels. Companies operating in electronic commerce arenas can utilize this data to better understand their markets and further optimize their businesses. Such organizations that invest in collecting the data, analyzing it, and acting upon it will have a significant advantage over those lagging behind.
Feedvisor’s intelligence and optimization platform includes three core pillars — pricing, advertising, and brand management. These pillars provide brands, retailers, large sellers, and private label businesses with the ability to optimize their Amazon operation from end-to-end, through data-driven insights and actions.
One of the core components in the platform is Feedvisor’s Trade Intelligence Network (TIN), which functions as a hub collecting commerce data from a myriad of sources, integrating them and processing them into meaningful insights and trends to be consumed by our algorithms and end users. Its main role is to provide a reflection of the market, by which we can more accurately assess our customers’ performance within their competitive space, provide valuable intelligence, and apply semi-automatic and automatic actions for improving their bottom line.
As a data powerhouse, TIN accumulates hundreds of millions of new commerce records each day, and altogether processes tens of billions of data points throughout the day — many of these in real-time — for providing rich and accurate conclusions in a timely fashion. The Network is a fundamental part of our system and is interconnected to everything we do across pricing, advertising, and branding.
A substantial part of the market knowledge generated based on TIN’s data is reached through AI and machine-learning techniques. Applying such techniques over the combination of operational data, marketplace data, and transactional data enables us to draw conclusive market insights and trends. We share several such examples below.
Marketplace Intelligence
As part of the marketplace intelligence package provided by Feedvisor’s platform, we utilize machine-learning based models developed over TIN for estimating the sales activity of competing products, brands, and sellers. Through this, Feedvisor customers can continuously measure their performance on a relative scale — in comparison to their competition.
By doing so, they are able to better understand their business performance in the market, accounting for seasonality and anomalous spikes in activity. Specifically, this provides the means to accurately assess the performance after initiating targeted promotions or advertising campaigns — i.e. we have not only improved the nominal value of our sales, but also the ratio between our sales and our competitors.
In addition, such quantitative intelligence can assist in determining our market share around a product or category and identifying areas in the market where we either are not operating in enough or at all. For example, if a customer is selling a shirt on Amazon, and the competitive arena is saturated with other shirt selling competitors, we will be utilizing TIN to understand how much share of the shirt market for this specific shirt our client has and how much more potential they may have in selling this shirt.
Pricing Optimization
One of Feedvisor’s main areas of differentiation in the market is around its repricing technology. As opposed to many other solutions which base their repricing technique on business rules generated by their users, Feedvisor applies algorithmic approaches for resolving this challenge. With just a minimal set of parameters provided by the user, such as cost structure and price boundaries, Feedvisor employs various machine-learning techniques for maximizing customers’ business results.
One of the key components in its repricing technology is a model acquired through deep learning, which assesses the competitive edge (positive or negative) a seller has over other competitors in the arena. This in time is used for determining the best price that can be placed per that seller and product at that time, for best optimizing the tradeoff between high margins on one hand and maintaining ranking, visibility, and sales on the other. This model is trained and based on hundreds of millions of snapshots of active arenas in the marketplace, across millions of products.
The knowledge deduced above is extremely important for selling on marketplaces such as Amazon, which ranks the offers available for a given product according to multiple variables, rather than just by price, such as fulfillment method, shipping time, seller rating, etc. On Amazon specifically, if a seller offers a product and their competitors are also selling the exact same product, they are all effectively fighting to acquire the Buy Box. The challenge there is to determine the highest price to win the Buy Box, given our customer’s characteristics, as well as their competitors’.
Connecting the Dots
Since its inception in 2014, TIN has been accumulating and analyzing vast e-commerce data from numerous sources. It can be viewed as a tremendous knowledge base accompanying our customers’ day-to-day operations. Together with Feedvisor’s proprietary algorithms and methods, it provides them with an unmatched advantage over their competition. In the future articles in this series, we will dive into additional topics and further demonstrate this advantage.
Learn what Feedvisor can do for your business.
When you partner with Feedvisor, you automatically receive access to our true, AI-driven technology and hands-on team of e-commerce experts. Contact one of our team members today to learn more about our end-to-end solution for brands and large sellers on Amazon, Walmart, and e-marketplaces.