Resources - Blog
Technology Series: The Application of AI in Advertising
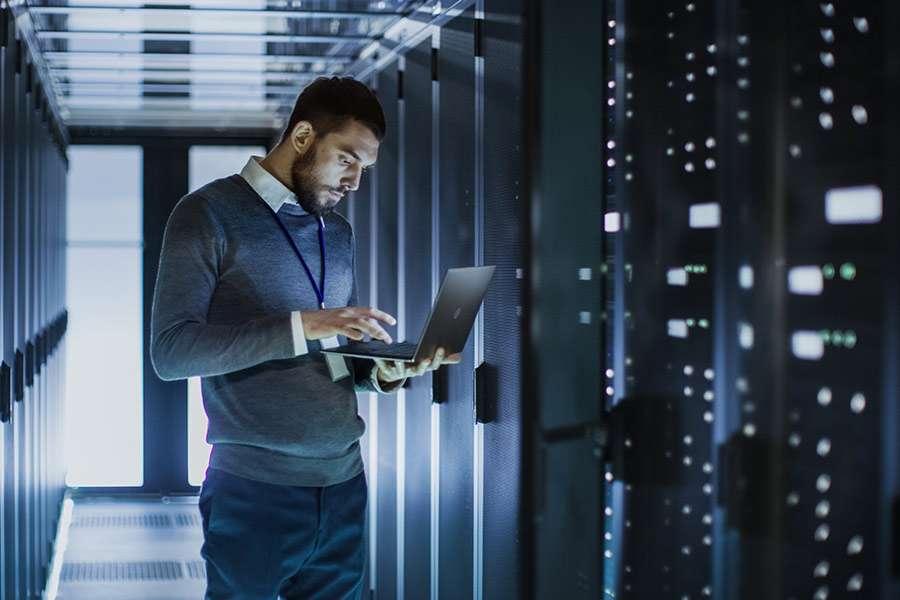
Stay on top of the latest e-commerce and marketplace trends.
The technology series focuses on Feedvisor’s AI-powered platform, data-driven intelligence, and machine-learning algorithms. The series will illustrate various ways in which our technology assists our customers in their e-commerce activities and challenges, with Amazon at the center.
The term “artificial intelligence” (AI) is used frequently today across a variety of industries, but what exactly is it? AI is a subset of computer science that focuses on machine-driven intelligence. Essentially, in AI-powered systems, machines mine, interpret, label, and react to data.
Very often, the machines functionally imitate cognitive practices normally attributed to humans, but the distinctive feature of AI-based systems is that the machine is not expected to follow a given “recipe” in order to find the right solution. Rather, it is expected to extract the optimal “recipe” on its own.
While today it seems like AI is present everywhere around us, for many years such systems were treated as an unfulfilled promise, as they often failed to provide significant improvements that justified the complexities associated with most of the algorithmic challenges it was aiming to mechanize.
The first domain in which intelligent systems showed tremendous improvements compared to traditional algorithms was the advertising industry, which ultimately led to the rapid growth of companies such as Google and Facebook, among others. In this blog, I will address some of the key reasons that advertising is a natural platform for AI-driven systems and what it takes to build an effective advertising engine.
4 Reasons Advertising Is the Ultimate Application of AI
1. The Methodology Fit
One of the most effective domains in AI is that of supervised learning. In supervised systems, the user provides historical events and the consequence of each event, known as train data. Based on this data, the system is then required to find an underlying set of rules that can provide an optimal explanation to the observed behavior and predict what the system response for future events will be.
This approach very adequately addresses the requirements from an advertising system, where we provide relevant information about historical events — such as advertised content, viewer details, clicks, and conversion results — and choose the strategy for future events based on the results of these events.
2. The Significant Amount of Data Available
There is one catch with the supervised learning approach — and with AI in general — and that is, in order to work well, it requires a significant amount of data. When attempting to learn the underlying rules that dominate a system, and assuming those rules are not trivial, an algorithm can easily jump to wrong conclusions that may fit the train data well but provide a poor description of reality, a phenomenon known as overfitting.
As an example, think about a baby that tries to understand which objects can move and which cannot. After observing a man and an apple, she may deduce that only objects with legs can walk. After an additional observation of a table, the conclusion will be that only objects with two legs, and not with four, can walk. If the next observation is a cat, the previous conclusion will also require modification, and so on.
Following the above scenario, in order to allow the algorithm to converge and offer an effective solution, we need to provide it with millions — and sometimes even billions — of examples. This is exactly the case in digital advertising, where billions of users respond multiple times a day to advertising triggers. The requirement for large data sets is also very beneficial: While a human observer will not be able to refine her conclusions after hundreds of observations, AI-powered algorithms keep learning and improving even after seeing billions of examples.
3. Computers Are Better Than Humans at Dealing With Details
Humans can cope better than computers with most of the tasks we face — at least for the time being — such as understanding complex situations, deciphering context, innovating, interpreting nuances, and so on.
On the other hand, computers are superior whenever it comes to processing large data sets, particularly those with a significant number of constraints or degrees of freedom. The rules that are governing advertising-related decisions are exactly this type. If you asked a human analyst to study and analyze the history of a system and define the rules that govern the system, he will manage, with great effort, to extract several rules.
On the other hand, a typical “neural net,” which is one of the most common designs for AI-backed systems, holds millions of parameters within the model that enable a much finer classification of events, therefore leading to much more accurate predictive capabilities.
4. Advertising Involves Data Analysis and Linguistics
One of the main challenges in designing a complex solution for a problem is the integration between different disciplines. Upon first impression, digital advertising is prone to integration issues, as it involves the analysis of large data sets, for which the effective tools are computational, and a deep understanding of text and language, a domain which is hard for traditional, non-adaptive algorithms.
One of the main breakthroughs in the AI domain was found to be extremely helpful here — the development of efficient natural language processing (NLP) frameworks. One of the great achievements of modern NLP, besides its notable efficiency, is that it knows how to translate linguistics into the classical optimization language of numbers and vectors by allowing an almost seamless connection between the different components of an advertising system.
The Constructs of an AI-Based Advertising Platform
In order to develop an effective, AI-driven platform, you must first begin with a strong understanding of the platform. For example, the rules, targets, and metrics for advertising on Amazon greatly differ from that of Google and Facebook. Approaches that may be successful on one platform may lead to suboptimal performance on the other and vice versa.
Additionally, you need relevant data, and a lot of it. In many cases, the requested data is publicly accessible and the harvesting task then focuses on identifying which data should be collected and managing the large data sets on an ongoing basis.
In other cases, the required data is not publicly accessible. On Amazon, for example, the most relevant data is the internal data that Amazon holds and shares only with the merchant, brand, or advertiser. For these advertising systems, gaining access to the desired data is as important as knowing how to manage the data flow.
You also need to make sure you have the appropriate infrastructures. While AI-driven applications are available today and building a neutral net is not as challenging as it was in the 2000s, managing very large data sets is still not a trivial task. It requires the ability to maintain up-to-date databases and data processes.
Final Thoughts
At Feedvisor, we have a very intimate acquaintance with the Amazon platform and many of the variables that influence success on the dynamic, hyper-competitive marketplace. As an industry-leading data company that strategically partners with the sellers and brands who operate on Amazon, we have a vast amount of transactional data that spans many products and categories and cannot be obtained via alternate methods.
Our research and development team functions as a line of defense to verify that we can modify and scale our platform according to the growth of the Amazon ecosystem, and are able to adjust our software and services according to our clients’ needs, as well as the challenges and opportunities Amazon presents to sellers and brands on its platform.
Learn what Feedvisor can do for your business.
When you partner with Feedvisor, you automatically receive access to our true, AI-driven technology and hands-on team of e-commerce experts. Contact one of our team members today to learn more about our end-to-end solution for brands and large sellers on Amazon, Walmart, and e-marketplaces.